Evaporation
Evaporation rate
Transient droplet heat-up is followed by steady-state evaporation, when droplet diameter decreases linearly in time according to the d2-law. The steady-state evaporation rate, λst, is defined as the slope of the linear decrease, depending on droplet size, ambient conditions, and thermophysical and transport properties of fuel vapor and surrounding gas. λst provides a computationally powerful tool for combustion chamber design, being a representative indicator for the intensity of droplet vaporization. Therefore, our group developed a calculation model, applicable for a wide range of ambient conditions for n-alkanes, highly relevant in combustion.
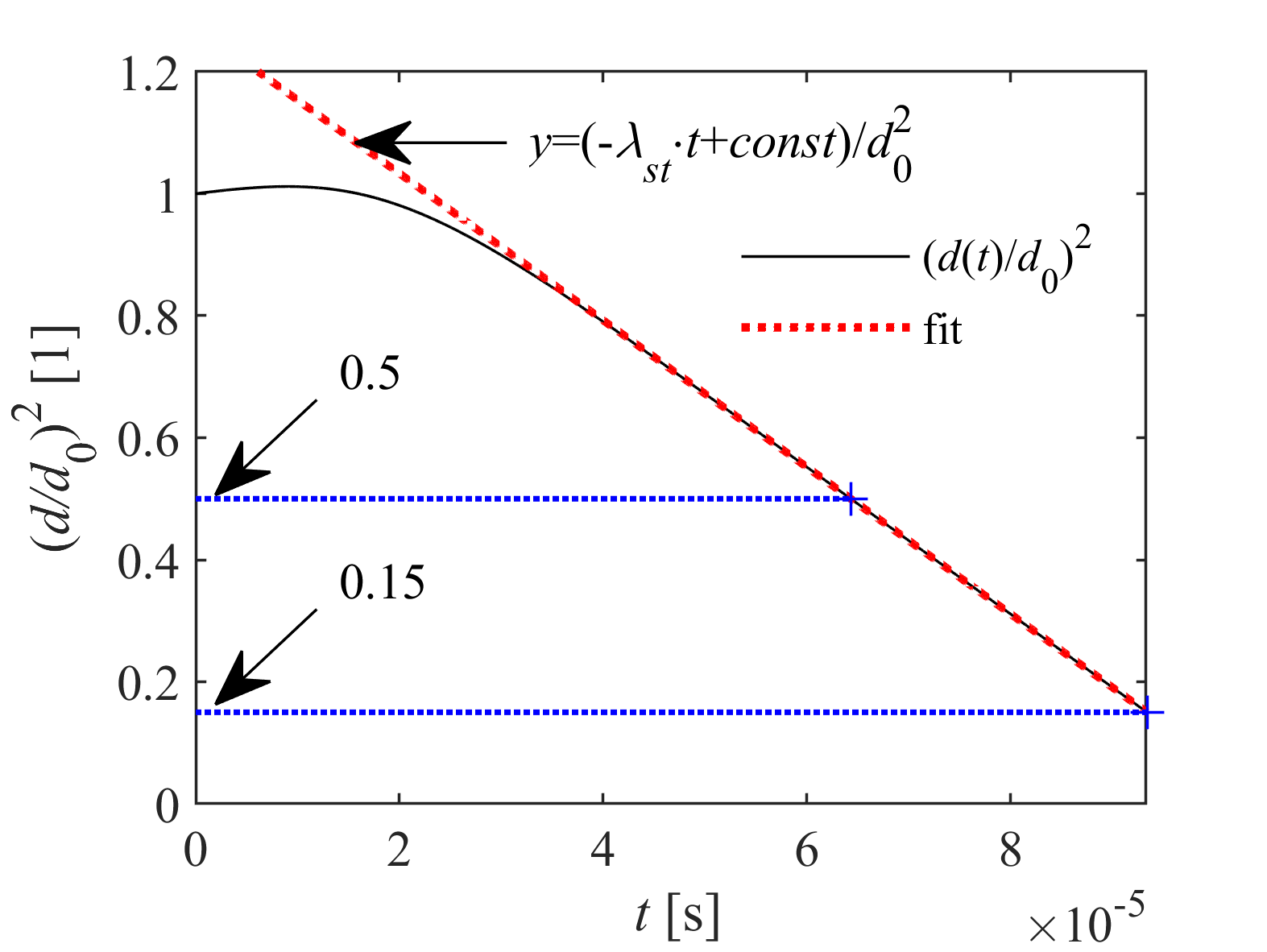
Model validation
The suspended droplet method, which is the typical experimental layout for single droplet evaporation measurements, is highly affected by thermal biases, encumbering model validation. The most important one is the additional heat conduction from the environment to the droplet via the quartz fiber suspension. A coupled numerical model was developed and validated by our group, accounting for the biasing effects in the thermal balance of the droplet and a parameter study was performed to evaluate the thermal behavior of the measurement setup for various liquid fuels. In this manner, we can identify the experimental parameters leading to marginal bias in order to facilitate the validation of our models.
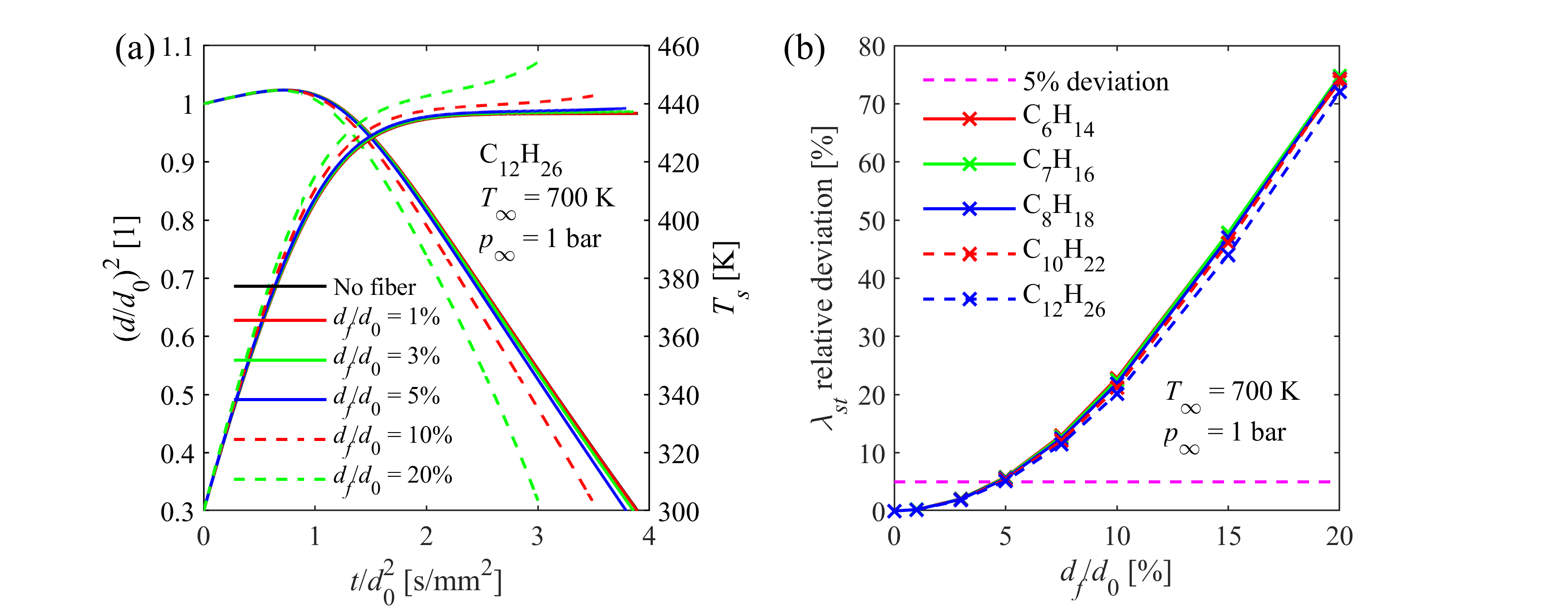
Model uncertainty
Spray vaporization shows significant sensitivity on thermophysical and transport properties of the fuel (e.g., D12, binary diffusion coefficient of vapor and ambient gas). Reliable reference data is often scarce in the literature, especially for renewable fuels, hence the properties are commonly patched with estimation models. However, the accuracy of these models are rarely published, thus the user of a commercial software code lacks information on their precision and effects on model uncertainty. Consequently, our group revised the available and widely-used estimation models for all the fuel properties required for modeling droplet vaporization and evaluated their accuracy for renewable fuels used in practical applications.
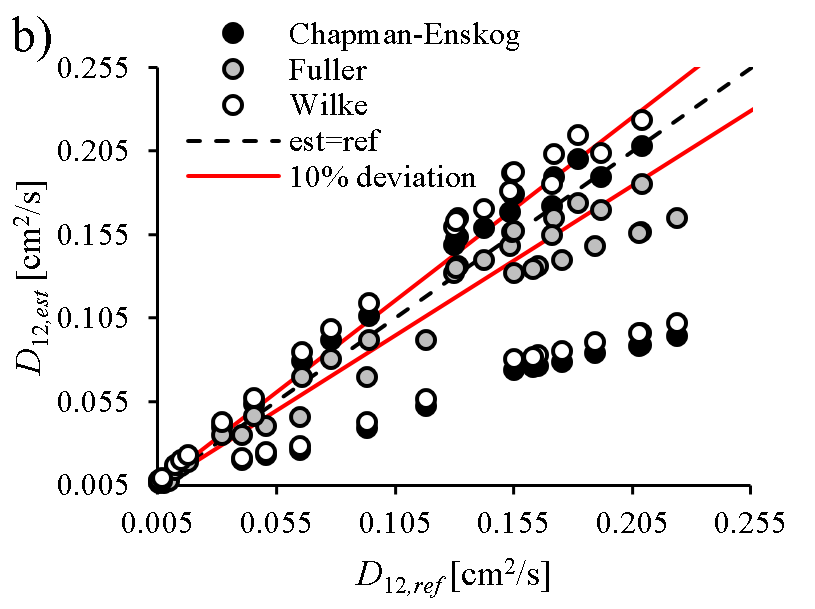
Fuel volatility
Droplet vaporization is highly affected by fuel volatility. Less volatile fuels require higher combustion air temperature for sufficient evaporation to provide stable combustion. Fuel volatility can be characterized by the distillation curve. Our group developed a modified ASTM D86 distillation apparatus with nitrogen flushing, applicable for biodiesel samples, as well, since thermal decomposition is prevented. Initial boiling point, TIBP, is determined from temperature data of properly placed multiple temperature sensors. One of our main goals is to relate volatility characteristics of different fuels to occurring flame shapes and combustion modes. Furthermore, we use the measured distillation curves for surrogate mixture formulation for modeling multicomponent droplet evaporation.
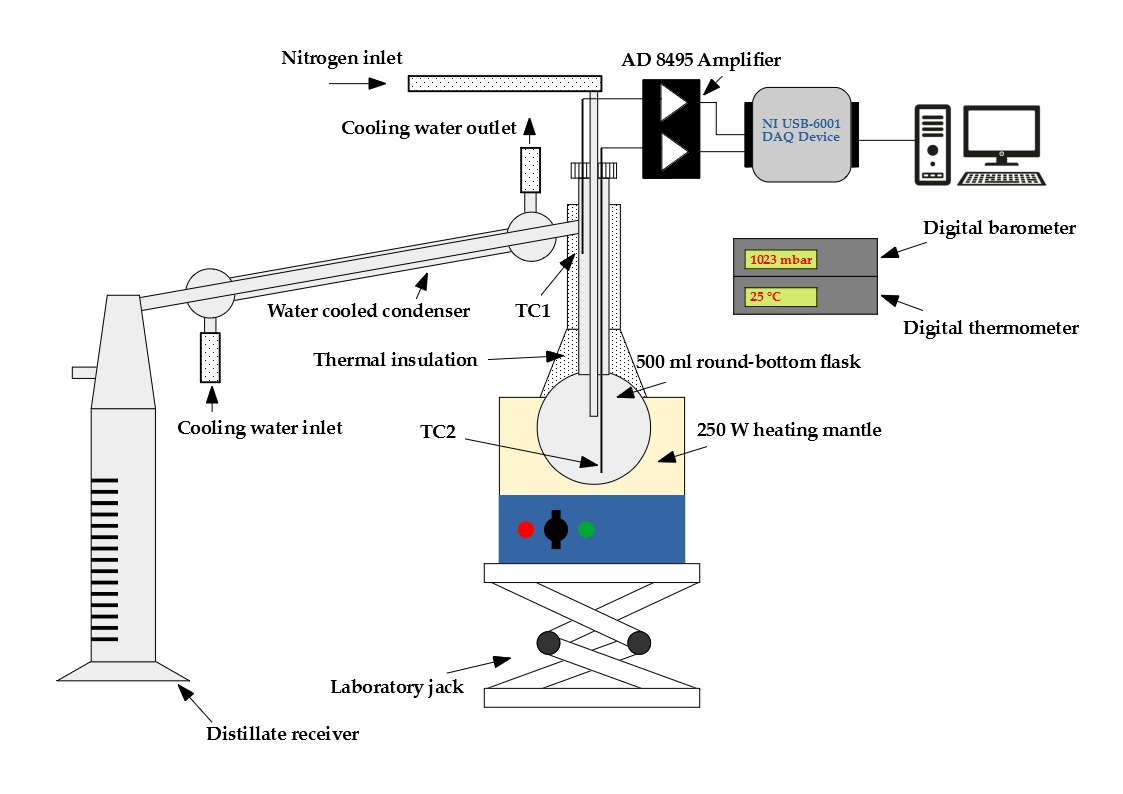
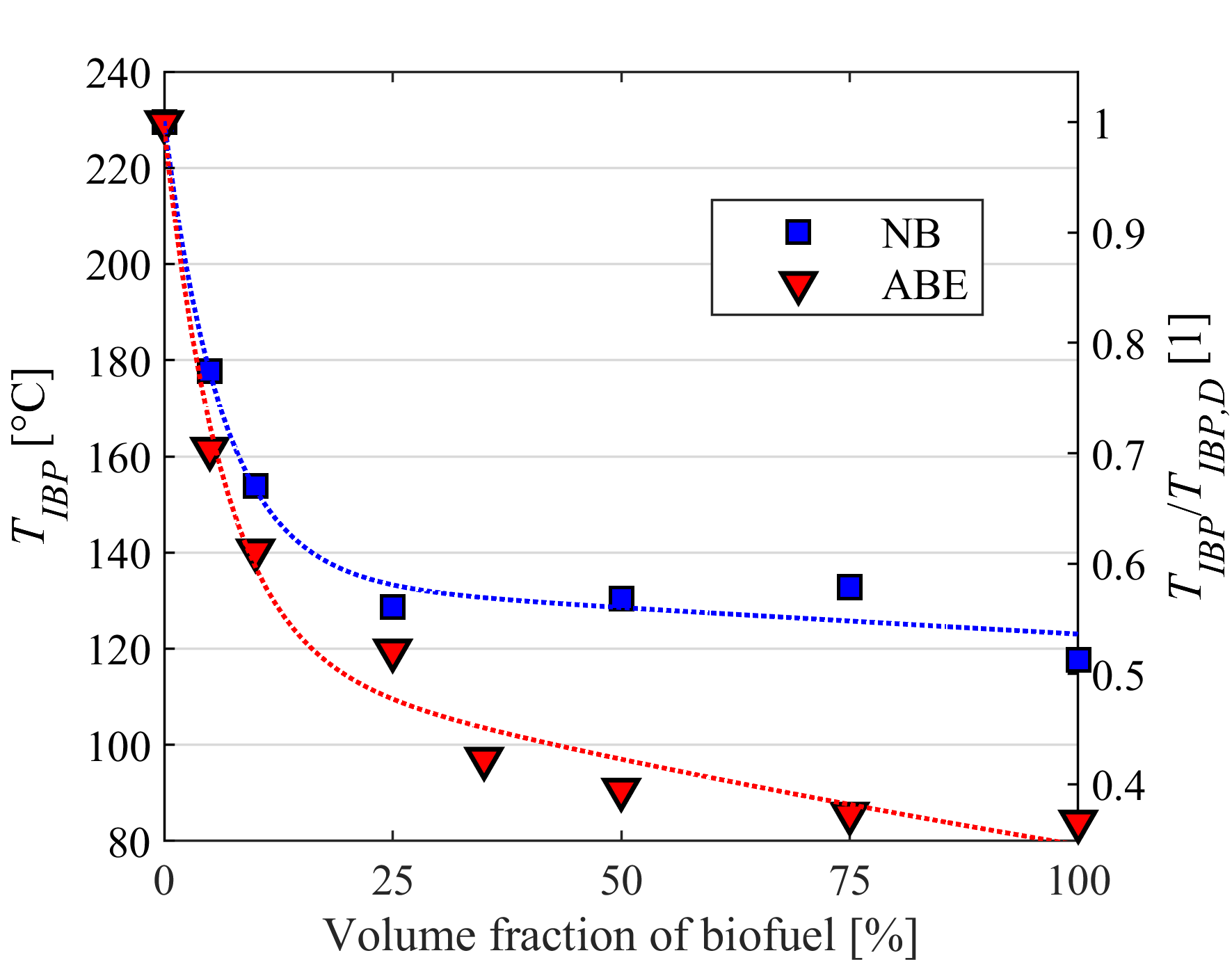
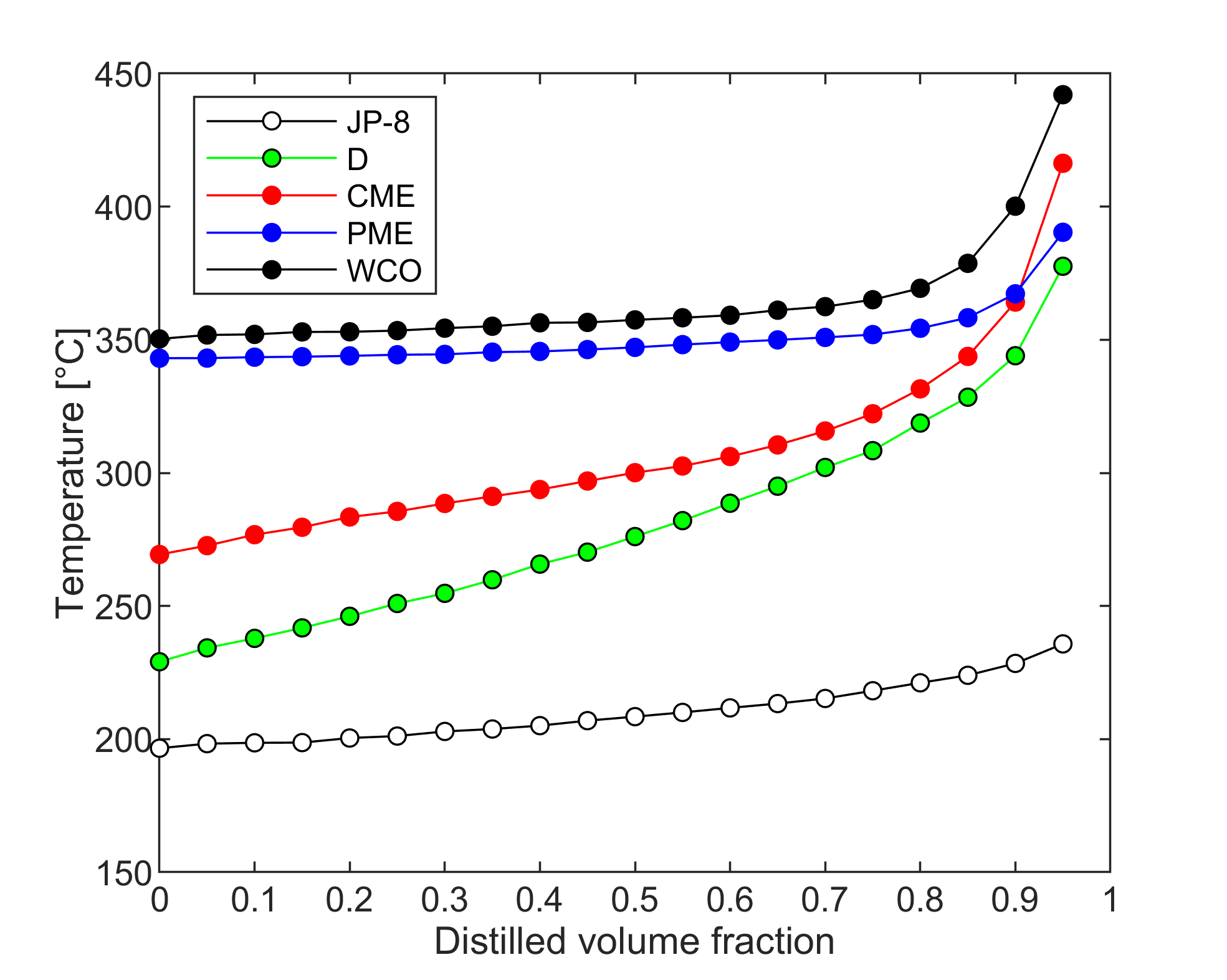
Surrogate mixtures
Modeling the combustion of commercial and renewable fuels used in practice requires multicomponent approach. However, considering the detailed composition is not computationally feasible due to the large number of equations to solve in large numerical grids. Furthermore, chemical kinetics data is often incomplete for certain compounds. Thus, surrogate mixtures with reduced number of components are applied in modeling environments, capable of reproducing physical and chemical behavior of the fuel. Our group focuses on atomization, evaporation, and chemical kinetics behavior, thus the composition of surrogate fuels are optimized based on measurement and reference data of density, viscosity, surface tension, flash point, and distillation curve, measured in our laboratory and also lower heating value, average molecular mass, and hydrogen-to-carbon atom ratio. We have optimized a surrogate mixture for diesel fuel, containing eight components, which can be directly implemented to modeling environments. Furthermore, we investigate the effect of component reduction on selected target properties in case of biodiesel samples to determine the minimum number of components that is required to reproduce fuel characteristics within the desired error. In this manner, we can formulate surrogate mixtures for biodiesel samples from various feedstocks.
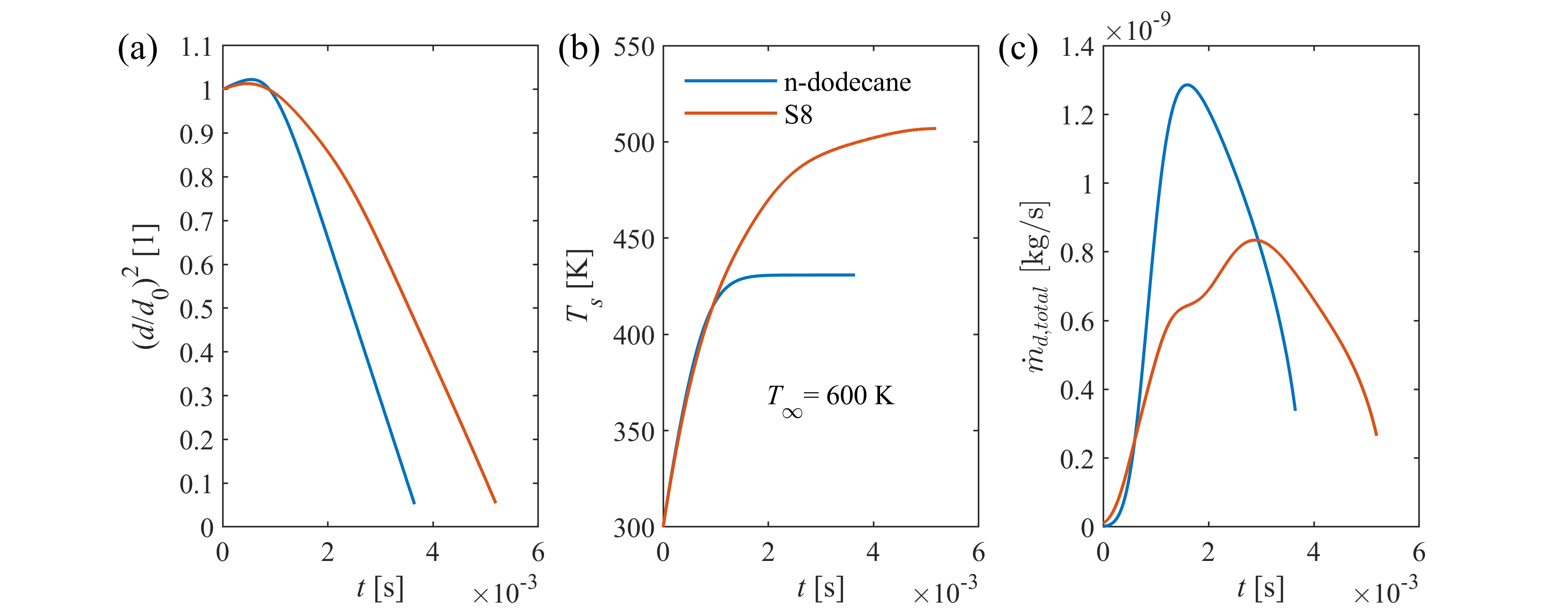

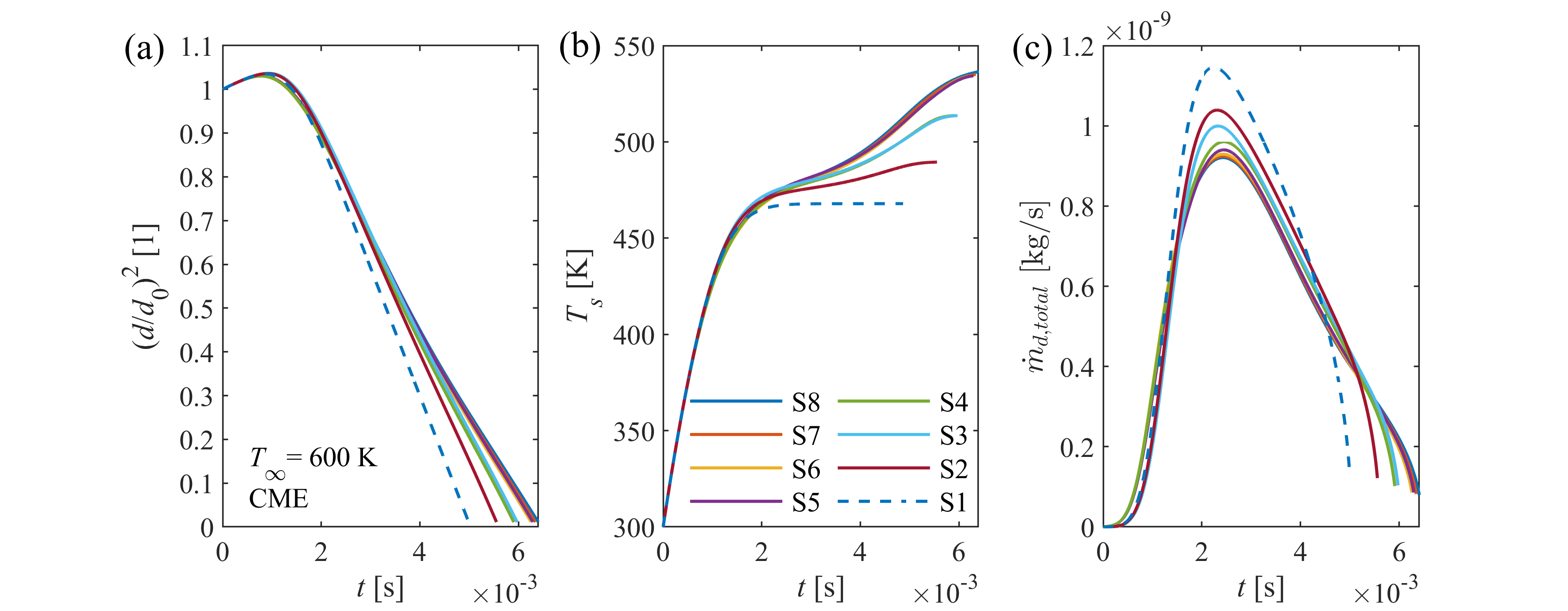